Becoming Open Minded About Open Source
By Troy Burmeister, Data Analyst & Claire Gilbert, Data Analyst, Gongos, Inc.
From software development to social networking, open source software powers big companies. Surprisingly, the people behind it don’t necessarily sit within the walls of Facebook, Google or Microsoft. They reside in thriving communities of active online users’ intent on fueling advances in data science, big data, and analytics. Perhaps more surprisingly, open source software is taking industries by storm, offering users—and the companies that employ them—a practical, if not obvious, alternative to their traditional analytical toolkit.
While launched in an academic setting, the acceleration of statistical software R for data wrangling and analysis inside corporations has given users access to features normally reserved for premium enterprise software. Most companies have gotten all too comfortable with setting aside budgets and fixed resources for this very purpose. But this legacy thinking comes at a cost.
The Limitations of Enterprise Software
The insights industry has long relied on proprietary software and technologies, and there’s been good reason for that. In an effort to power work streams that extract insights from structured data, enterprise software systems offer proven solutions, customer support, and are accountable to upgrades and development. Yet, this “black box” mentality keeps insights organizations from truly advancing in a landscape increasingly sculpted by open source releases—leaving little room for innovation. If you take a step back, it’s easy to see the irony in this. Corporations have an increasing appetite for technology-driven breakthroughs, yet the majority of market research-based analysis is neatly packaged within the confines and constraints of boxed software.
The Promise of Open Source
To truly understand open source software’s appeal to a data scientist, one needs to see it from the vantage point of the people that created this ecosystem: the developers and contributors (those building the tools) as well as the users (those putting them to use).
The Developer
|
The User
|
It’s the hybrid incarnation of the “Developer/User” that makes the open source world both distinct and alluring. Users that see a need within the community morph into the role of developer, and developers transform as users of their own product. As a consequence, developers are motivated to continually update and offer their expertise, while users readily find answers to their questions. This coexistence not only fuels further reliance on the open source community, but leads to the development of tools for nearly every data challenge. In the article above, Google’s chief economist deftly refers to this phenomenon as “standing on the shoulders of giants.”
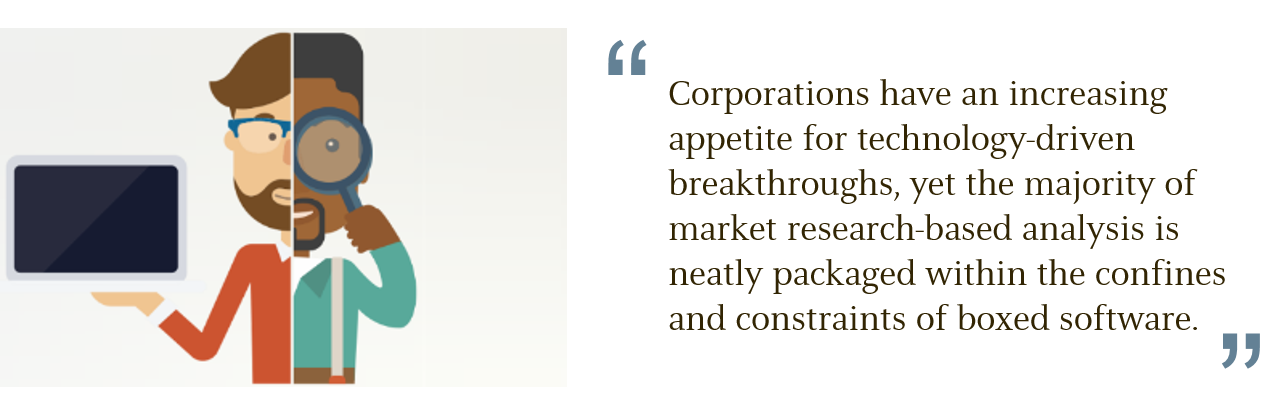
Beyond standard data management, this powerful ecosystem leads to highly customizable solutions and real-time software innovation, giving organizations the aptitude to consistently remain at the forefront of cutting-edge methodologies. More specifically, it empowers data scientists with: the ability to develop and leverage programs that respond to highly targeted needs not widely available through traditional software; shorter time span from ‘idea’ to ‘usable feature’; and a captive community of “co-creators” invested in creating new tools that enable big and ‘small data’ analytics capable of adapting to the needs of virtually any industry.
Open Source in the Insights Industry
The proficiency cycle of the “Developer/User” has enabled data scientists to further push the limits of both longstanding and burgeoning market research techniques. It is driving more consumer-friendly data collection, while delivering richer business-minded insights on the backend. As the new kids on the block, data scientists are bringing both the skillsets and mindsets to adapt to and solve newer, more complicated problems—and organizations that employ them are taking notice.
An Organization Embracing Open Source
Recently, an analytic challenge brought these benefits to light for a client organization. Working with data collected in a path-to-purchase study, there were struggles on several fronts. Restructuring individual touchpoints from a survey into a cohesive path was inadequate, if not impossible, in our standard ‘boxed’ software. Moreover, there was a strong desire to employ a clustering algorithm to understand how paths grouped together—and the ‘boxed’ software didn’t provide a sound solution for clustering binary data. Leveraging Python allowed us to quickly wrangle our data into a usable format. We then turned to R, finding several packages of newer clustering algorithms designed specifically for modeling binary data. The instant download and testing of these packages enabled us to expedite an unparalleled approach that empowered the client to activate on and infuse new intelligence throughout the organization.
As analysts flock to the open source community for answers, they will constantly be met with a morphing and growing library of software solutions to mine and analyze data for new insights. The collective learning environment perpetuated by the “generosity” of this community empowers researchers—and data scientists—with new levels of statistical confidence when consulting with organizations. This momentum will not only allow for the experimentation necessary for the insights industry to remain relevant, but will continue to evolve organizational intelligence.